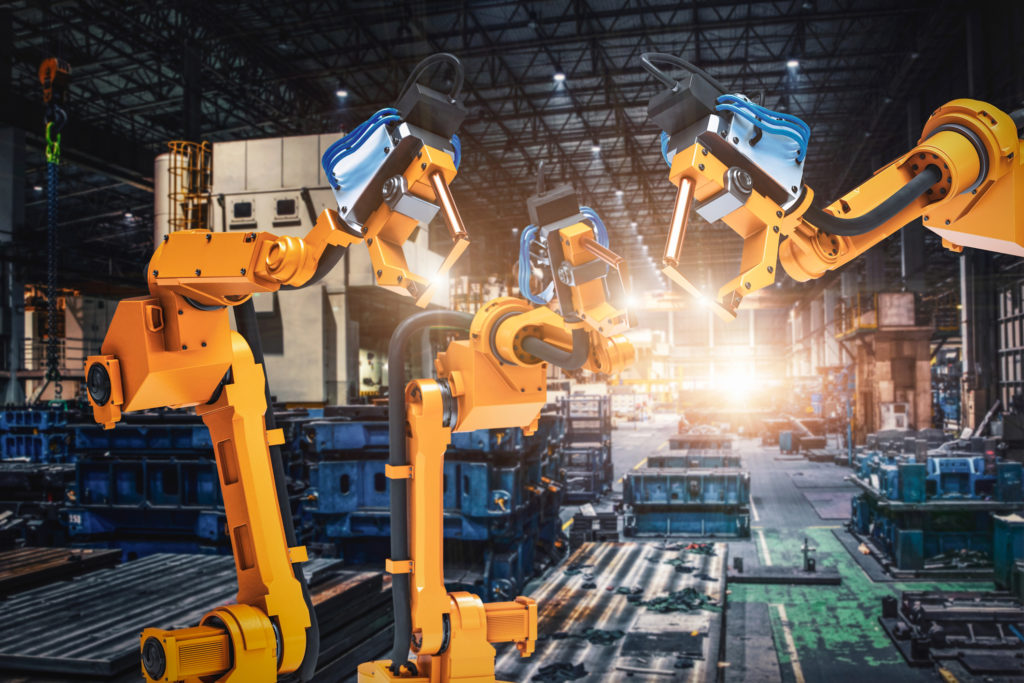
1952 saw the first computer program that could learn as it ran. It was a game which played checkers and was created by Arthur Samuel.
Fast forward to 2019 and the usage of machine learning (ML) and artificial intelligence (AI) has accelerated to real-world use cases that can be applied to modern-day business problems.
Here are just a few of the ways that machine learning and AI improves our quality of life on a daily basis:
- Receiving a movie or song suggestion while browsing video or music streaming services like Netflix, YouTube, and Spotify.
- Using smart devices, such as the Nest thermostat, to determine your home’s optimal temperature settings when you’re home and away.
- Google provides suggestive “predictions” based on the initial keyword(s) that you type into the search bar.
- Machine learning and its subset, AI, have been deployed to assist companies with solving legacy problems related to IT systems (e-mail spam, threat detection, and mitigation) as well as physical security (theft and shrink reduction).
- Document recognition and compliance to check signatures on thousands of documents—a process that takes humans hours or days to complete.
- Image analysis using ML and AI is showing promise in the detection of cancerous tumors and is being used to diagnose and determine action to mitigate risk.
- Chatbots, or automated “Level 1” support, provides customer service support without human intervention.
What is Machine Learning and Artificial Intelligence?
AI is a broad term that refers to the doctrine or study of training systems to perform tasks in a more efficient manner than humans can execute.
Machine learning is more nuanced and is often referred to as a “subset” of AI. Machine learning speaks to the systems, processes, and specific frameworks that are required to perform a task. This enables technology to actually “learn” and potentially provide value. The goal of machine learning is to ingest data input such as logs and images with the intent of learning things from that data.
Room for opportunity, and what enterprises are doing – by the numbers
I recently came across a Forbes article that polled C-level executives on the state of AI propagation in their respective enterprises. Poll results demonstrate that these leaders want to implement some form of AI in their respective line of business.
- 47 percent of business executives have embedded at least one AI capability in their business processes.
- 21 percent say their organizations have embedded AI in several parts of the business.
- The Forbes article also cited a McKinsey study which found that 30 percent of businesses surveyed are piloting AI.
- According to a RELX Group survey, 55 percent of government officials are aware of AI but say it is not being utilized, while 37 percent of surveyed officials are utilizing AI.
- According to PricewaterhouseCoopers, only 15 percent of enterprises have appointed a single enterprise-wide AI leader while 3 percent said they were not sure who was in charge of AI, and none of the respondents said there was a single C-level executive who was in charge of AI at their firm. 24 percent said their enterprise-wide AI efforts were being led by an AI “center of excellence.”
First engagements with clients concerning machine learning and AI – what we have encountered
Most of our clients are generally in the discovery phase. This is when an organization studies how machine learning and AI can help streamline business processes and provide a relevant return on investment (ROI).
Unfortunately, we’ve also learned that many clients don’t know where to start when classifying what data is relevant to implement an AI strategy that aligns with their business needs. And there are often challenges with how they utilize data with an AI/machine learning framework in mind when the identified business process is data rich.
We also see businesses trying to operationalize an AI framework from an IT perspective before clearly identifying the appropriate use cases. We have engaged with clients that want to start this conversation using the same approach they would in sizing a “traditional” IT workload.
What OEM is the market leader, from a server perspective?
What GPU should I utilize?
Can we virtualize this workload?
What are your other clients utilizing to manage this infrastructure?
Will the new AI platform that I am evaluating integrate with my current network/storage topology?
These questions are fundamentally relevant but are not necessarily the most pertinent at the onset of the AI conversation. The success of any ML/AI deployment heavily relies on conversations with both business and technology leaders. It is essential to understand the entire business before discussing the speeds and feeds of any technology that will eventually follow.
Personnel, the AI practice, and the ability to execute
Finally, it’s important to mention the overall importance of skillsets that are typically required to deploy a successful AI infrastructure. In most cases, these skillsets are radically different from what enterprises have traditionally needed.
“Typical” legacy application deployment relies heavily on the systems administration and application development skillset. The machine learning and AI deployment shifts that focus from a primarily infrastructure-centric discussion to a more business-centric/data science approach.
This shift requires a new set of skills for success. Machine learning and AI professionals are typically more data driven and often hold advanced degrees in mathematics and/or computer science. They are typically subject matter experts in the area of statistics, data mining, and programming.
These roles typically include:
- Data scientists, who are proficient with the knowledge of extracting data and who can interpret that data using tools and frameworks commonly found in the ML/AI stack. Data scientists spend a majority of their time collecting, preening, and massaging data to eventually drive actionable results. Data scientists are typically not primarily interested in the hardware technology, infrastructure, or day-to-day operations of a given deployment. Their primary goal is to evaluate data and provide meaningful insights.
- Data engineers typically have knowledge specific to infrastructure and data architecture. Data engineers are often involved with the methods, tools, and infrastructure required to discover, extract, convert, and move data to its respective AI platform.
These data-centric skillsets are becoming increasingly difficult to find as the adoption of AI increases in the enterprise.
CBTS and our value add with machine learning and AI
The challenges specific to AI adoption quickly become apparent as these disciplines gain traction in the enterprise.
CBTS brings multiple aspects of value to your AI deployment based on our experiences with clients in multiple industries.
Perhaps you are just beginning to examine how an AI framework can bring value to your enterprise. Maybe you require assistance with finding qualified data science and engineering resources in a very competitive workforce. And there’s always a need to understand the right approach when selecting the appropriate infrastructure to deploy and operationalize your AI effort.
You can be confident that CBTS has the expertise to assist you wherever you are in your AI and machine learning journey.