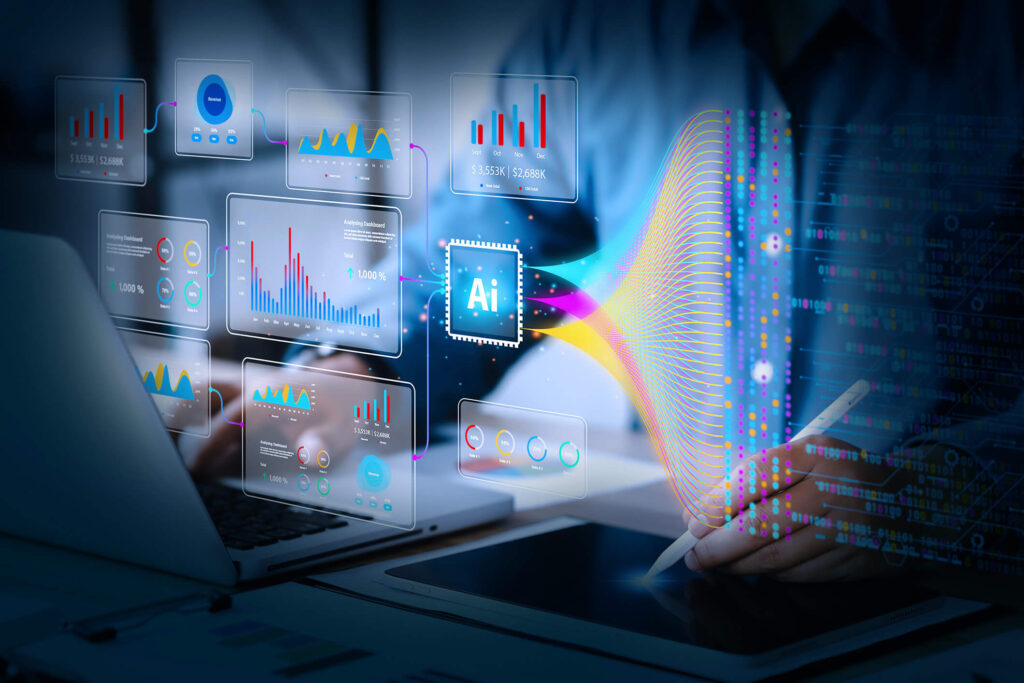
At first glance, artificial intelligence (AI) seems like the perfect solution for managing large amounts of data. Properly implemented AI can standardize, aggregate, and organize raw data while mining for the business insights that drive profit. However, the risks of AI implementation at the enterprise scale come with two categories of risk:
- Modernizing infrastructure that can keep up with AI.
- Data management that is secure, efficient, and compliant with privacy laws.
This blog examines how organizations can address these risks and benefit most from the AI revolution.
What is modern data management?
Data management encompasses methods of collecting, aggregating, securing, and analyzing data. Modern data management incorporates the latest technology (like AI) into data management processes and frameworks.
Traditional data management | Modern data management |
Structured data | Data ranging from unstructured to structured |
On-premises data center | Leverages cloud or hybrid cloud computing |
Limited by capital expenditure investments | Uses an operating expense model to scale |
Manual processes, aggregation, and analysis of data | Utilizes advanced algorithms and automation |
Limited integration options | Advanced APIs and microservices |
Storage restricted by onsite hardware | Cloud-based databases, data lakes, data warehousing and data meshes. |
Struggles to scale with growing data volumes. | Easily scales to accommodate increasing data volumes and business needs. |
Lacks a comprehensive data governance framework. | Implements robust data governance policies to ensure compliance and quality. |
Learn more: How embracing the latest AIOps technology can improve customer experience
AI and data management: Inherent risks
Data management risks
Security is a critical risk in data management. Modern technology, such as AI and machine learning, may access sensitive data in new ways, creating inadvertent leaks or new weak points for bad actors or over-privileged users to exploit.
Data quality is also an issue for AI. Faulty or inaccurate data limits even the world’s best large language models (LLM) and can compromise the quality of an AI’s analysis.
AI and compliance
Privacy
AI models are designed to learn by analyzing data. However, if the data used for training is private, sensitive, or regulated, it raises privacy concerns. Large amounts of data can be used to identify specific individuals. AI models that learn from proprietary customer data put enterprises at risk of sensitive information leaks.
LLMs must be constantly monitored as they learn, as each new interaction creates a potential for inadvertent exposure. If a customer withdraws their data from a company (an option required by GDPR) and has already used that data to train a model, it may need to be dismantled and retrained without the withdrawn data.
Despite the risks, organizations of all sizes prioritize AI deployment to reap the benefits of better analytics and insights, customer behavior analysis, improved app performance, and a host of other competitive advantages.
Also read: The significance of AI: Explore the future of collaboration and communication
Infrastructure challenges of AI
Organizations attempting to deploy AI across existing infrastructure may be in for an unfortunate surprise. AI takes a massive amount of compute power to function efficiently. In addition, enterprises seeking to implement AI into their processes successfully must also contend with the following:
- Storage – Traditional storage options may lack the speed and agility needed for AI and modern data management priorities. Many organizations are seeking on-prem storage to address AI security concerns, but this option may not scale as quickly as cloud storage or Infrastructure as a Service (IaaS) solutions emerge to meet the demands of AI.
- Networking – Storing the data needed for AI is one issue—transferring, accessing, and analyzing that data is quite another. Organizations need high bandwidth and low latency to keep up with the lightning-fast processing demands of AI.
Learn more: CBTS launches AI-powered Network as a Service in partnership with Juniper Networks
Infrastructure solutions for modern data management
The efficacy of AI solutions largely depends on whether they have a specialized infrastructure to support them. Many such initiatives fail due to inadequate infrastructure that cannot efficiently handle the complexities of machine learning workloads. Building an infrastructure that is intended for AI can be a challenging task. It requires a significant investment of time and money, as well as in-depth knowledge of AI.
AI-specific infrastructure
IT providers are beginning to offer “AI-ready” or “GPT-in-a-box” solutions to get organizations up and running with AI solutions as fast as possible. AI-specific hardware is fast, highly scalable, and changeable—three attributes addressing the previously discussed performance, networking, and storage issues.
Edge computing
Edge computing is a processing method that brings computation and data storage closer to where it is needed. This proximity significantly reduces latency, increasing the speed of AI applications and use cases. This immediacy is particularly useful for AI applications, where real-time processing and decision-making are often critical.
Cloud AI
Cloud-based AI infrastructure is a game-changing approach to accessing and utilizing business AI capabilities. The business size does not matter; this model is designed to provide access to the latest AI technology without requiring substantial on-premises capital expense investments.
By using cloud computing, businesses can easily access vast computational resources on-demand, scaling up or down as needed. This flexibility not only makes AI more affordable but also promotes greater flexibility and agility. Additionally, the cloud-based model ensures that AI tools are available anywhere, anytime, breaking down geographical barriers and creating a more global workspace.
Managing the transition to enterprise AI
Modern data management begins with a secure, fast, and scalable modernized infrastructure, which is vital to effectively executing AI at every level of the technology stack. However, choosing the correct infrastructure approach to implementation that meets your unique situation can be challenging. AI capabilities are still developing. Should your organization invest in an on-prem system that prioritizes security? Or should your organization utilize a scalable cloud or hybrid cloud model that transforms CapEx into monthly operating expenses? One thing is sure—a trusted advisor will enable your organization to obtain the results you need.
AI is not the first digital revolution CBTS has helped our clients navigate. From cloud computing to secure access service edge, we’ve helped our customers keep pace with radical changes to the technology landscape to ensure they stay competitive, profitable, and efficient. Speak with one of our experts today.
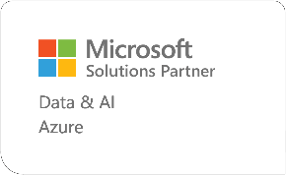